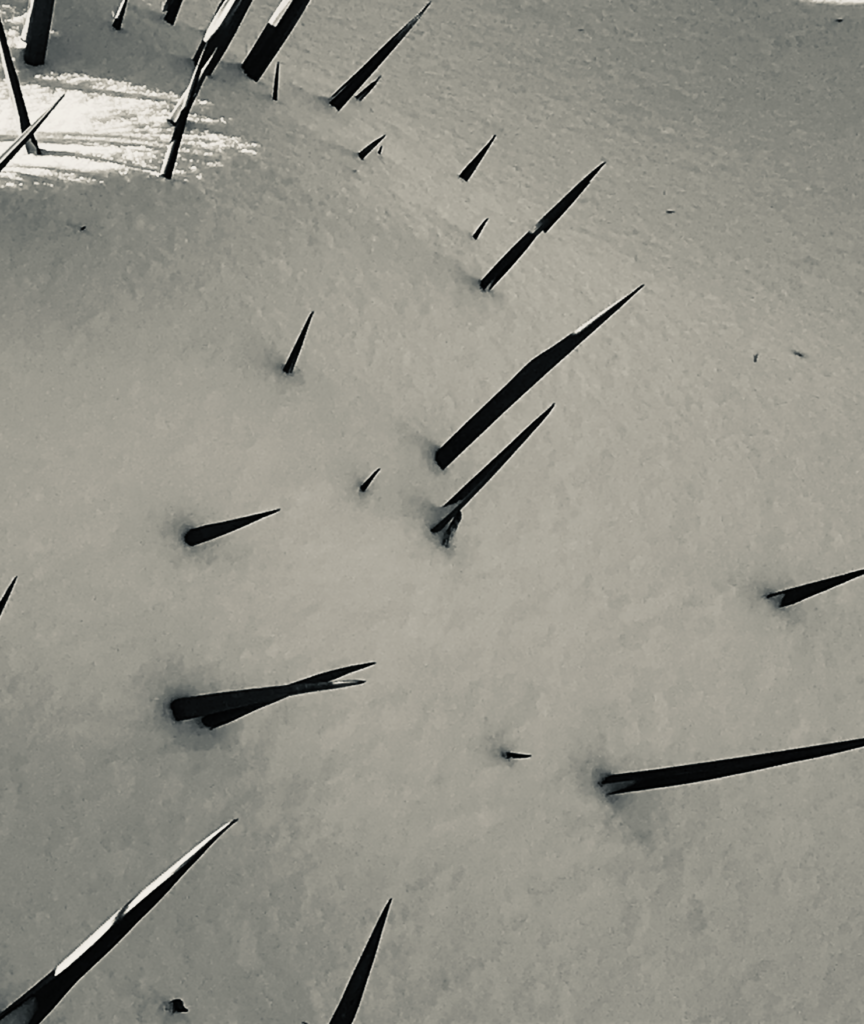
I know that a neural network is not a network of neurons, but for the rough scaling and order-of-magnitude arguments, is it legitimate to equivalence a synaptic connection with a weight in a deep network such as a transformer?
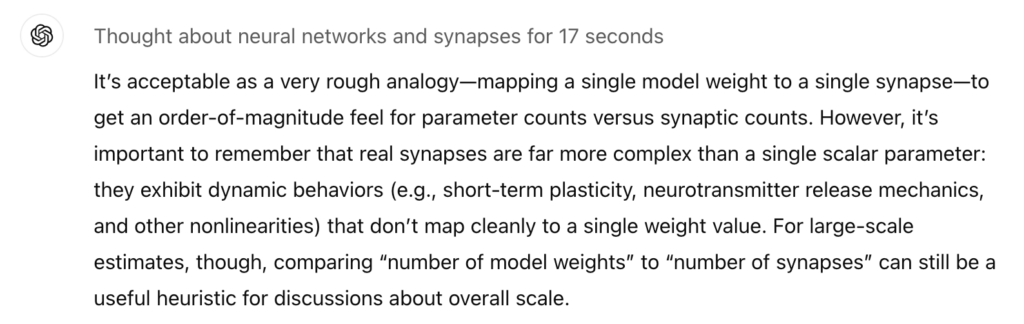
That’s all the sign-off I need to get going.
In a post toward the end of last year, I used synapse-to-weight equivalence to argue that GPT-2 was accomplishing the rough computational equivalent of a fruit fly brain. Next, queue some requisite marveling at the gigantic difference in inference-time energy costs. Note, however, that scientific bloggers run the continual risk of excessive chewing over the same tired themes (IMC-1, anyone?) I won’t burden everyone with more moralizing.
What is clear, however, is that the massive AI-infrastructure build-out that dominates the news these days is making an implicit bet on the perpetuation of extreme computational energetic (as well as likely algorithmic) inefficiency.
Human brain has 100 trillion synaptic connections. Using the equivalence rule of thumb, that makes it somewhere on the order of 100x the size of GPT-4. Let’s consider the energy cost of pre-training. Training the brain uses 100 watts for 25 years. Rounding up a bit, that’s a 1e+18 erg energy budget. GPT-4 was estimated to consume 50 GW-hours to train. That’s 2e+21 ergs for 1% of the model complexity, so it’s a factor of roughly 200,000x less efficient. The immediate implication is that the current transformer architecture, and the current architectural paradigms are nowhere near optimal. If NVDA is to retain their outsize valuation, they’ve got to innovate radically, and probably quickly.
My guess is that somebody else, however, somebody not currently on the radar, will get there first.