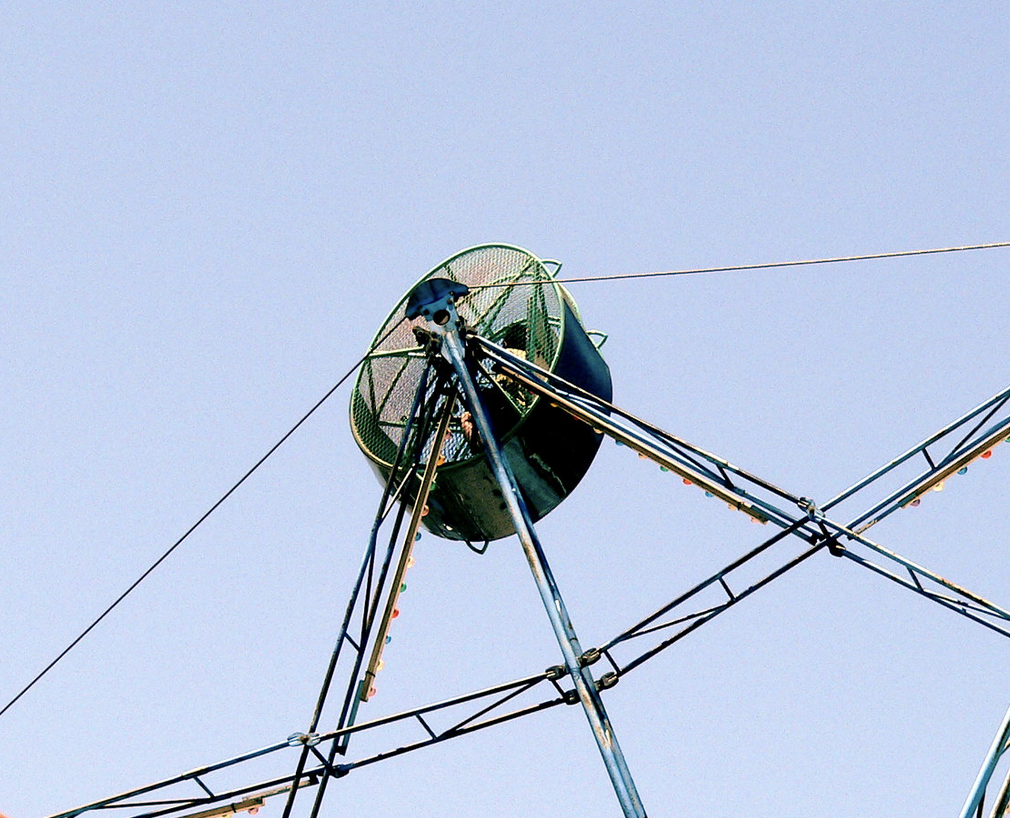
It’s not hard to find grumbling on Hacker News regarding the venture firm a16z in general, and their (now-going-on-a-year-out-of-date) AI Canon in particular. It’s a reading list for those scrambling to get up to speed in the brave new world of generative AI. Read definitively not quixotic. The one item that I’ve consistently found most useful from the canon is Simon D. M. Prince’s Understanding Deep Learning, the latest draft of which is hosted on GitHub. The chapter on Transformers is very clearly written.
Speaking of Brave New World, I was reflecting that the a16zai cannon blasts out items of tone ranging from just-the-facts dry to business-focused dry. It could maybe use some novels at the end. Which ones to choose?
I am thinking Mary Shelley’s Frankenstein. Despite a publication date of January 1 1818, it’s a total on-trend allegory for the current moment. Same goes for C.S. Lewis’ That Hideous Strength. I have read and savored the chapters where Mark Studdock is the central character upwards of twenty times. They are perfectly marbled with deep truths regarding motivations and academia and organizations. (Note that I’ve read the chapters taking place at St. Anne’s on the Hill and involving Ransom et al. precisely once). I’m also thinking that Bradbury’s Martian Chronicles aren’t necessarily about Mars.
Cervantes’ Don Quixote is one book that certainly wouldn’t appear in even the most liberal extension of a16z’s AI canon. Interestingly, though, it begins with lines in an obscure composition style known as versos de cabo rato, and these are oddly relevant to a jet fresh discussion of language models:
If to reach goodly read-
oh book, you proceed with cau-,
you cannot by the fool-,
be called a stumbline nin-,
But if you are too impa-
and pull the loaf untime-
from the fire and go careen-
into the hads of the dim-
you’ll see them lost and puzz-
through they long to appear learn-
Handing the rest of the post over to a generative pre-transformer,
Conceptual Illustration of Branching Token Predictions
Given the line “If to reach goodly read-“, the model might consider a range of possible continuations like:
- “ers” (probability: 30%)
- Continuation: “If to reach goodly readers, one must…“
- “ing” (probability: 25%)
- Continuation: “If to reach goodly reading, it’s essential…“
- “able” (probability: 20%)
- Continuation: “If to reach goodly readable content…“
- “ily” (probability: 15%)
- Continuation: “If to reach goodly readily, prepare…“
- “er” (probability: 10%)
- Continuation: “If to reach goodly reader, engage…“